Our lives have been transformed by artificial intelligence (AI), which has shaped industries and transformed the way we interact with technology. From virtual assistants to self-driving cars, AI is revolutionizing various sectors. In this blog, we will delve into the basics of AI, explore machine learning and deep learning, and discuss the different types of AI.
What is Artificial Intelligence?
Artificial Intelligence refers to the development of intelligent machines capable of performing tasks that normally require human intelligence. It includes speech recognition, problem-solving, decision-making, and learning. The goal of artificial intelligence is to simulate human intelligence in machines, enabling them to analyze large amounts of data, recognize patterns, and make informed decisions.
Strong AI (General AI):
Strong AI, also known as General AI, refers to AI systems that possess human-level intelligence across a wide range of tasks and can understand, learn, and apply knowledge in a manner similar to humans. The goal of strong AI is to create machines that can think, reason, problem-solve, and exhibit consciousness. In addition to being able to understand the world, they would also be capable of being creative and possessing common sense.
The development of strong AI is a complex and ambitious goal that researchers have been striving to achieve for many years. It requires the ability to replicate human cognitive abilities, such as understanding language, learning from experience, and engaging in abstract thinking. Achieving strong AI would mean that machines could surpass human intelligence and perform any intellectual task with the same level of proficiency.
However, despite significant advancements in AI, achieving strong AI remains elusive. The challenges lie in replicating the intricacies of human intelligence, including emotions, intuition, and the ability to comprehend complex concepts in various domains. Ethical considerations also come into play when developing strong AI, as its capabilities could potentially raise questions about consciousness, responsibility, and the impact on human society.
Weak AI (Narrow AI):
Weak AI, also referred to as Narrow AI, is the most prevalent form of AI that we encounter in our daily lives. It refers to AI systems designed to perform specific tasks within a limited domain, focusing on excelling at one particular function. These systems are trained and optimized to perform well in a narrow scope and lack the ability to generalize their knowledge to different tasks or domains.
Narrow AI systems are designed to tackle specific problems efficiently. They rely on predefined rules, algorithms, and large datasets to make decisions or perform tasks. Examples of narrow AI include voice assistants like Siri or Google Assistant, recommendation systems used by online platforms, image recognition software, and autonomous vehicles.
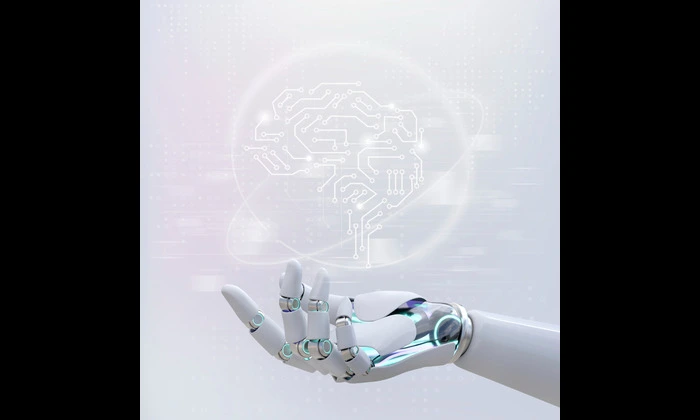
Unlike strong AI, narrow AI does not possess consciousness or the ability to reason beyond the specific task it is designed for. These frameworks succeed inside their characterized limits yet miss the mark on more extensive mental capacities related to human insight. However, narrow AI has demonstrated tremendous value in various industries, driving significant advancements and practical applications.
Machine Learning
Machine Learning is a subset of AI that focuses on enabling machines to learn from data without explicit programming. Instead of being explicitly programmed, machine learning algorithms use patterns and statistical models to learn and improve from experience.
- Supervised Learning: In supervised learning, the machine learning model learns from labeled data, where inputs and desired outputs are provided. The model learns to make predictions or classify new, unseen data based on the patterns it learned from the labeled examples.
- Unaided Learning: Solo learning includes preparing AI models on unlabeled information. The model expects to find stowed-away examples or designs inside the information with practically no particular result names. Bunching and dimensionality decreases are normal undertakings in solo learning.
- Support Learning: Support learning includes preparing specialists to go with choices in a climate to expand a prize. The specialist learns through experimentation, getting criticism as remunerations or punishments. Over the long haul, it learns the ideal moves to make in various circumstances.
Deep Learning
Deep Learning is a subfield of machine learning that focuses on using artificial neural networks to model and understand complex patterns. These neural networks, inspired by the human brain, are composed of multiple layers of interconnected nodes (neurons). Deep learning algorithms automatically learn hierarchical representations of data by processing vast amounts of labeled or unlabeled data. This enables deep learning models to excel in tasks such as image recognition, natural language processing, and speech synthesis.
History of AI
1950s-1960s: The Birth of AI and Early Exploration
- In 1950, British mathematician and computer scientist Alan Turing proposed the “Turing Test” as a criterion for determining machine intelligence.
- In 1956, the field of AI was officially established during the Dartmouth Conference, where the term “Artificial Intelligence” was coined.
- Early AI research focused on symbolic reasoning and problem-solving, leading to the development of early AI programs such as Logic Theorist and General Problem Solver.
1960s-1970s: Symbolic AI and Expert Systems
- Symbolic AI, also known as “Good Old-Fashioned AI” (GOFAI), dominated the field during this period. Researchers used symbolic manipulation and logical rules to represent knowledge and perform reasoning.
- The development of expert systems, which used specialized knowledge to solve complex problems, gained prominence. MYCIN, an expert system for diagnosing blood infections, was a notable example.
1980s-1990s: Knowledge-Based Systems and Neural Networks
- Research shifted towards knowledge-based systems that utilized large knowledge bases and rule-based reasoning to mimic human expertise.
- Neural networks experienced a resurgence, thanks to advancements in computing power and the development of backpropagation algorithms. This led to breakthroughs in pattern recognition and speech processing.
Late 1990s-2000s: Machine Learning and Big Data
- Machine learning gained traction as a dominant subfield of AI. Algorithms such as support vector machines, decision trees, and Bayesian networks were widely used for tasks like data mining and predictive analytics.
- The advent of the internet and the availability of vast amounts of data led to the emergence of big data analytics, which played a crucial role in advancing AI algorithms and applications.
2010s-Present: Deep Learning and AI Expansion
- Deep learning, fueled by the development of deep neural networks and the availability of massive datasets, revolutionized AI. Breakthroughs in image recognition, natural language processing, and autonomous driving were achieved using deep learning techniques.
- AI applications expanded across various domains, including healthcare, finance, robotics, and virtual assistants. Companies invested heavily in AI research and development, leading to advancements in areas like reinforcement learning and generative models.
- Ethical concerns and discussions surrounding AI, such as bias, privacy, and job displacement, gained prominence, leading to the formulation of guidelines and regulations.
The history of AI showcases the progression from early theoretical concepts to practical applications, driven by advancements in computing power, data availability, and algorithmic innovations. As AI continues to evolve, the future holds the potential for even more groundbreaking developments that will shape our world in significant ways.
Conclusion
In conclusion, the history of AI is a fascinating journey that spans several decades. It has evolved from its conceptual beginnings to a field of practical applications that impact various aspects of our lives. Over time, AI has witnessed significant advancements, driven by breakthroughs in computing power, algorithmic innovations, and the availability of vast amounts of data.
From the early exploration of symbolic AI and expert systems to the resurgence of neural networks and the rise of machine learning, AI has experienced remarkable milestones. The advent of deep learning, with its ability to process complex patterns and make breakthroughs in image recognition, natural language processing, and other domains, has propelled AI to new heights.
AI has found its way into numerous industries, transforming healthcare, finance, robotics, and many others. It has become an essential tool for data analysis, decision-making, automation, and personalized experiences. However, along with these advancements come ethical considerations, including issues of bias, privacy, and job displacement, which need to be addressed responsibly.
As we look to the future, the potential of AI appears boundless. Continued research and innovation in areas such as reinforcement learning, generative models, and explainable AI promise even more exciting developments. Striking a balance between leveraging the power of AI and addressing the associated ethical and societal implications will be crucial as we move forward.
Ultimately, AI continues to shape our world, offering immense opportunities and challenges. With ongoing advancements, it holds the potential to transform industries, solve complex problems, and augment human capabilities. The history of AI serves as a testament to human ingenuity and the relentless pursuit of creating intelligent machines that can revolutionize the way we live, work, and interact with technology.
FAQ about Artificial Intelligence
What is Artificial Intelligence (AI)?
Artificial Intelligence refers to the development of intelligent machines that can perform tasks that typically require human intelligence, such as decision-making, problem-solving, learning, and perception. AI aims to simulate human intelligence in machines to analyze data, recognize patterns, and make informed decisions.
What are the different types of AI?
AI can be categorized into two types: Narrow AI (Weak AI) and General AI (Strong AI). Narrow AI is designed for specific tasks within a limited domain, while General AI aims to possess human-like intelligence across a wide range of tasks and domains.
What is the difference between Machine Learning and Deep Learning?
Machine Learning is a subset of AI that focuses on enabling machines to learn from data without explicit programming. It involves algorithms that can learn patterns and make predictions or classifications based on the data. Deep Learning is a subfield of Machine Learning that utilizes artificial neural networks with multiple layers to model and understand complex patterns, achieving exceptional performance in tasks like image recognition and natural language processing.
How does AI learn from data?
AI systems learn from data through various techniques. In supervised learning, models are trained on labeled data, where inputs and desired outputs are provided. Unsupervised learning involves training models on unlabeled data to discover hidden patterns or structures. Reinforcement learning involves training agents to make decisions in an environment and learn through trial and error, receiving feedback in the form of rewards or penalties.
What are some practical applications of AI?
AI has found applications in numerous fields, including healthcare (diagnostics, drug discovery), finance (fraud detection, algorithmic trading), autonomous vehicles, natural language processing (voice assistants, chatbots), image and speech recognition, recommendation systems, and robotics.
0 Comments